AI Image Recognition: The eDiscovery Feature You Didn’t Know Existed
Use AI Image Recognition to Unlock Hidden Opportunities In Your Visual Data by ProAI Sep, 2023
The idea that the photos we share are being collected by companies to train algorithms that are sold commercially is worrying. Anyone can buy these tools, snap a photo of a stranger, and find out who they are in seconds. But researchers have come up with a clever way to help combat this problem.
All of that sounds cool, but my business is online, so I don’t need an IR app, you might say. If you have a clothing shop, let your users upload a picture of a sweater or a pair of shoes they want to buy and show them similar ones you have in stock. If we did this step correctly, we will get a camera view on our surface view. First off, we will list which architecture, tools, and libraries helped us achieve the desired result and make an image recognition app for Android.
What is the level of interest in Image Recognition Software?
In the case of single-class image recognition, we get a single prediction label with the highest confidence score. In the case of multi-class recognition, final labels are assigned only if the confidence score for each label is over a particular threshold. Facial recognition is another obvious example of image recognition in AI that doesn’t require our praise.
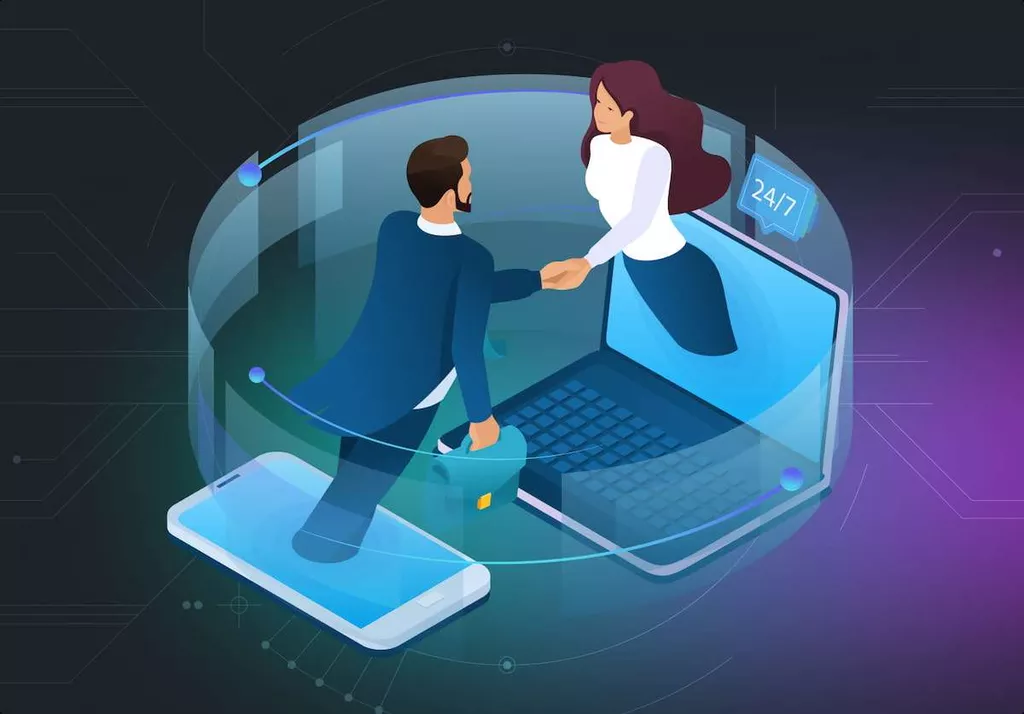
Face recognition is becoming a must-have security feature utilized in fintech apps, ATMs, and on-premise by major banks with branches all over the world. AI-based image recognition can be used to help automate content filtering and moderation by analyzing images and video to identify inappropriate or offensive content. This helps save a significant amount of time and resources that would be required to moderate content manually. Optical Character Recognition (OCR) is the process of converting scanned images of text or handwriting into machine-readable text.
Stock Photo Search
So far, you have learnt how to use ImageAI to easily train your own artificial intelligence model that can predict any type of object or set of objects in an image. AI-based image recognition can be used to detect fraud by analyzing images and video to identify suspicious or fraudulent activity. AI-based image recognition can be used to detect fraud in various fields such as finance, insurance, retail, and government. For example, it can be used to detect fraudulent credit card transactions by analyzing images of the card and the signature, or to detect fraudulent insurance claims by analyzing images of the damage. Data collection requires expert assistance of data scientists and can turn to be the most time- and money- consuming stage.
Now, let’s explore how we utilized them in the work process and build an image recognition application step by step. To train a computer to perceive, decipher and recognize visual information just like humans is not an easy task. You need tons of labeled and classified data to develop an AI image recognition model. Unlike ML, where the input data is analyzed using algorithms, deep learning uses a layered neural network. The information input is received by the input layer, processed by the hidden layer, and results generated by the output layer. This clustering algorithm runs periodically, typically overnight during device charging, and assigns every observed person instance to a cluster.
AI songs flood ingsocial media – AlgoaFM News
The main objective of image recognition is to identify & categorize objects or patterns within an image. On the other hand, computer vision aims at analyzing, identifying or recognizing patterns or objects in digital media including images & videos. The primary goal is to not only detect an object within the frame, but also react to them. We use the most advanced neural network models and machine learning techniques. Continuously try to improve the technology in order to always have the best quality. Each model has millions of parameters that can be processed by the CPU or GPU.
These tools provide improved situational awareness and enable fast responses to security incidents. In some applications, image recognition and image classification are combined to achieve more sophisticated results. While metadialog.com image recognition and image classification are related, they have notable differences that make them suitable for distinct applications. The goal is to train neural networks so that an image coming from the input will match the right label at the output.
Read more about https://www.metadialog.com/ here.